In a world where AI systems deduce and learn from examples, data is everything. There is a noticeable shift in the focus of labour required when working with contemporary algorithms; from a strong emphasis on code under the old, hard-coded approaches to datasets assembly in machine learning models.1 Formats, sizes, diversity, and variety; all of it matters. In architecture, digital tools are already the industry norm, and so are virtual environments and immersive experiences. Machine learning is slowly entering the field through optimisation models and generative plug-ins. It is, however, fundamental to understand the role of data in AI to realise its full potential in the design process and, as such, to redefine the architectural practice within the changing landscape of non-human cognition and its spatiality discussed in the previous two columns Unlearning architectural history and Cyberspace.
It is fundamental to understand the role of data in AI to realise its full potential in the design process and, as such, to redefine the architectural practice within the changing landscape of non-human cognition and its spatiality.
In her book Contagious Architecture (2013), Luciana Parisi points out that all modern algorithms are inherently dependent on data, whether they compute or produce it. "Algorithms are (thus) actualities, defined by an automated prehension of data in the computational processing of probabilities."2 How can we, architects, feed into this process of automatic prehension? The answer can perhaps be found by looking at historical examples of well-established compression methods for translating design information into organised datasets; architectural notation.
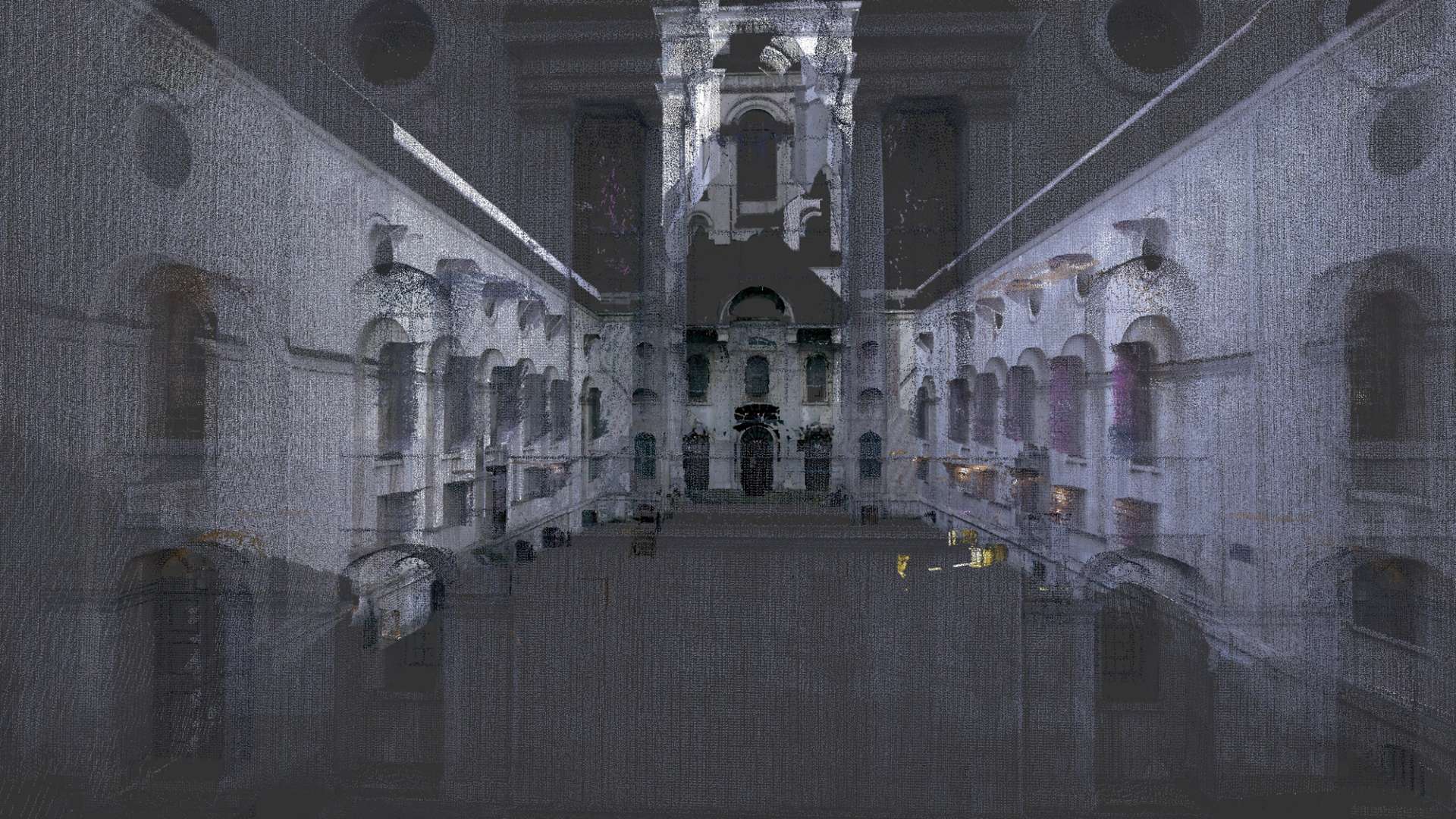
Point cloud scan of the Christ Church Spitalfields in London, exterior. ©Gosia Starzyńska, 2022.
As the designers of the process that translates spatial ideas into buildings, architects are ideally placed to negotiate the impact of the non-human cognitive systems over the physical reality by redefining what notation of the future should entail.
In his book The Alphabet and the Algorithm (2011), Mario Carpo traces the emergence of modern architectural notation back to the renaissance and Leon Battista Alberti's De Re Aedificatoria.3 Alberti aspired to create a system that unambiguously prescribed quantitative design data of a built form he had envisaged. On the one hand, information produced by the architect had to convey the maximum amount of data to avoid any uncertainty on site. On the other, if the documents were too excessive, their quantity could, in itself, create confusion. Carpo argues that, in that sense, architects did not produce buildings but information that described the design via different formats without the need for the architect to be on site.4 As the designers of the process that translates spatial ideas into buildings, architects are ideally placed to negotiate the impact of the non-human cognitive systems over the physical reality by redefining what notation of the future should entail.
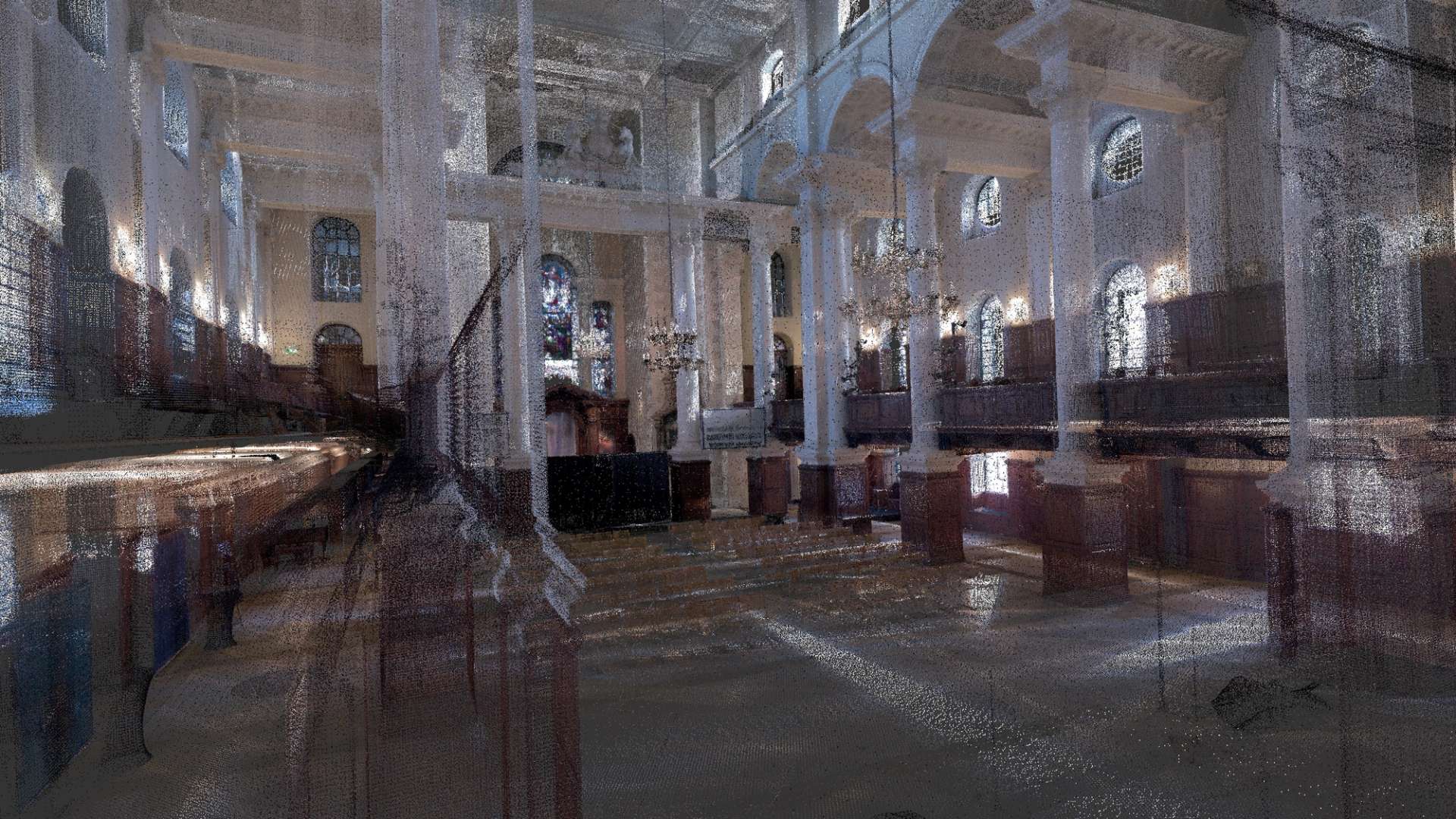
Point cloud scan of the Christ Church Spitalfields in London, interior. ©Gosia Starzyńska, 2022.
Introducing those processes to architectural practice will become an integral part of human-machine collaboration, an equivalent of the old tradition of identifying relevant views and scales that best describe a specific design aspect.
Carpo notes that the emergence of digital technologies has made the conventional problems of scaled representation irrelevant to digital modelling. "Contemporary CAD-CAM technologies have simply obliterated the notional gap that for centuries kept design and construction apart."5 The relevance of scale, however, has shifted from drawing scale to the model's level of accuracy and detail. Depending on the design stage or construction contract, information would be gradually layered, resolving build-ups and details at increasingly more granular scales.6 If we look at the practice of formatting data for machine learning purposes, increasing its complexity in that way is in direct opposition to how data needs to be cleaned to fit models.7 In that sense, it is impossible for an algorithm to study a digital model accounting for all its elements simultaneously. Extracting normalised datasets and singling out the aspects of the building that are relevant to the task will be key. We already see architectural practice shifting from annotated drawings to rule-based notation. For example, the 'if-then' statements are integral to schedules and fundamental for generative design.8 The next step will augment this process even further by automatically deriving those rules from the raw data itself. Introducing those processes to architectural practice will become an integral part of human-machine collaboration, an equivalent of the old tradition of identifying relevant views and scales that best describe a specific design aspect.
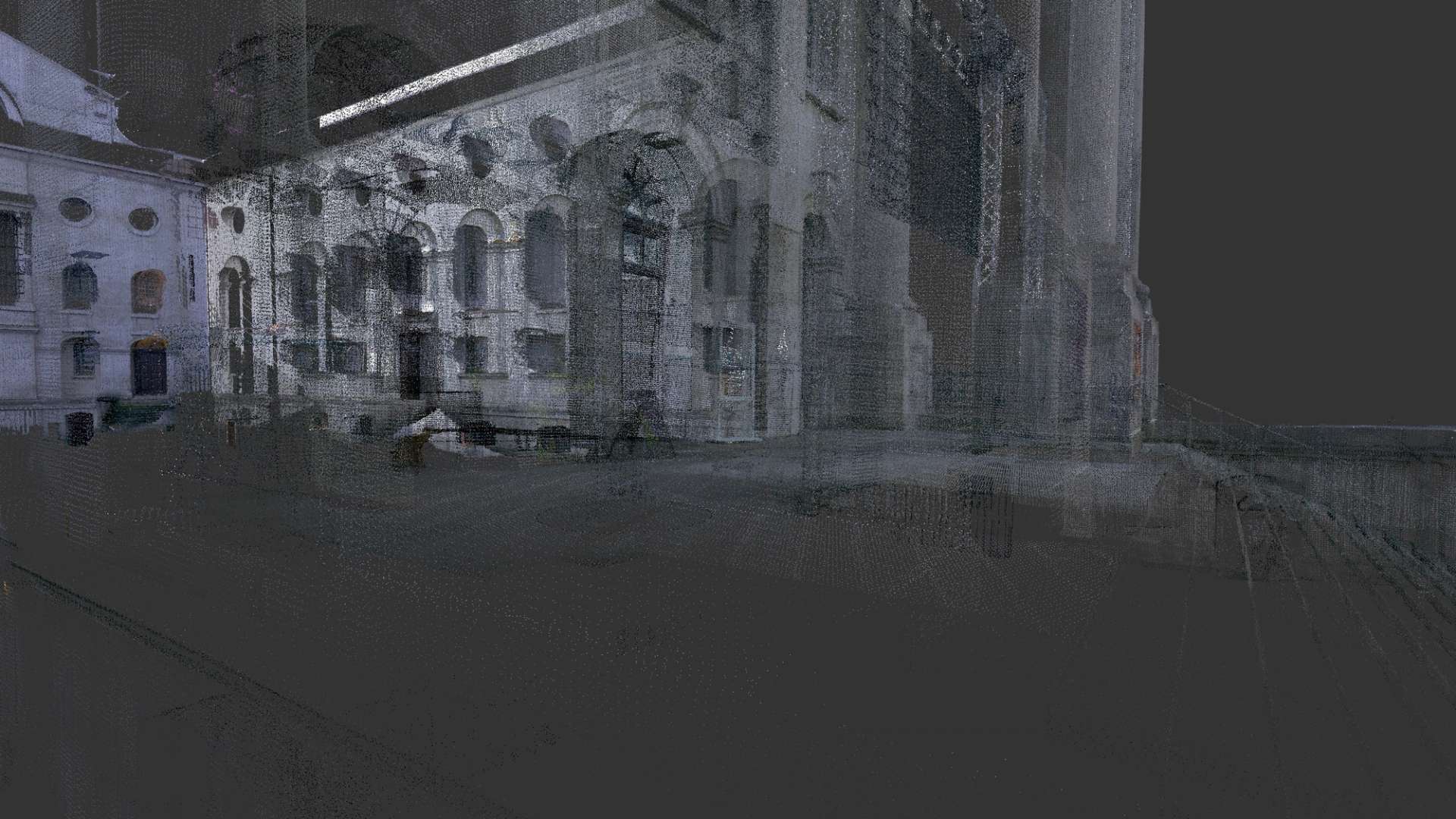
Point cloud scan of the Christ Church Spitalfields in London, exterior. ©Gosia Starzyńska, 2022.
While the traditionally incompatible sets of notational information and scales became unified under the digital model, a new type of variety was introduced; formats. Whether point clouds, depth maps, RGB-D, voxels, or meshes, each has its own aesthetic and means of denoting spatiality. Ways for manipulating space for each file type, in turn, can influence the practice of designing. Carving is different from assembling or pulling things apart. Those aesthetic nuances become amplified when documenting existing buildings through digital formats as computational cost competes with details. The tension between the compression and accuracy of data is apparent. For example, a point cloud model of Christ Church Spitalfields in London (figures 1-4), a sparse collection of points, illustrates a very different experience of space to the real-life building by Nicholas Hawksmoor.9 While each detail of the church is documented through a corresponding point at 10mm intervals, without the solidity typical to other formats such as mech or voxel, the building's geometries are layered in front of each other creating vistas unique to the format, not to the building.
The tools of our practice are evolving, but the nature of it remains unchanged.
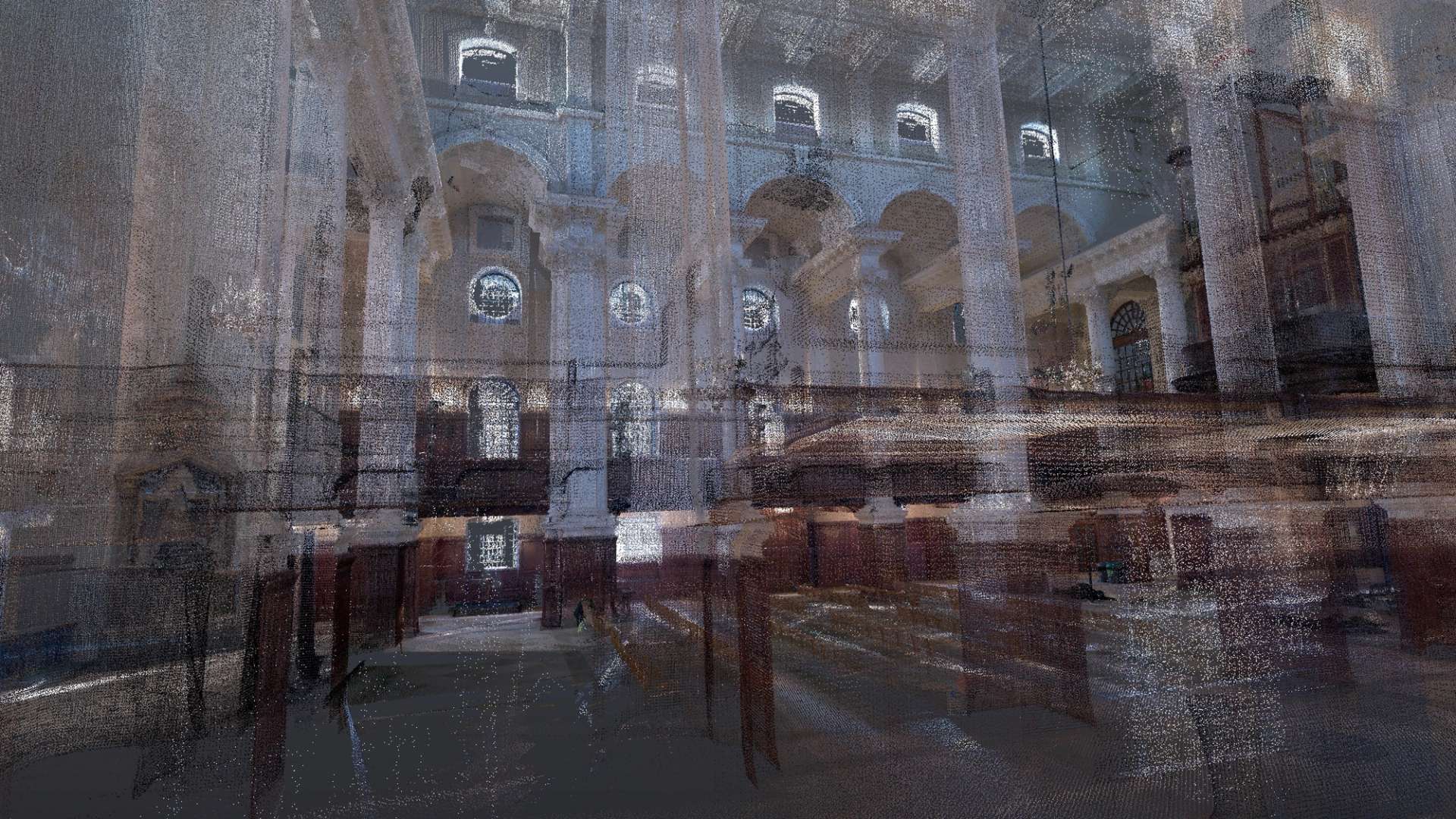
Point cloud scan of the Christ Church Spitalfields in London, interior. ©Gosia Starzyńska, 2022.
The tools of our practice are evolving, but the nature of it remains unchanged. Architects have always worked with compression; whether through scale or reduction in dimensionality, our role was to develop a representational model of the building that balances the two: accuracy and data size. Scale or two-dimensional drawings aimed to retain the maximum information at the minimum processing cost. In that sense, this balance between data diversity and its volume has always been at the heart of design practice since Alberti. For architects, curating data will be a continuation of the centuries-long quest for the notational system that best captures the essence of the building without overwhelming the reader.
Architects have always worked with compression; whether through scale or reduction in dimensionality, our role was to develop a representational model of the building that balances the two: accuracy and data size.
Read the entire "Architecture of Machine Dreams" column by Gosia Starzyńska.
Bio
Gosia Starzyńska is an architect, an educator and a current researcher at the Laboratory for Design & Machine Learning at the Royal College of Art in London. She is also an associate lecturer at Oxford Brookes University where she leads machine learning and data-driven design teaching curriculum for the Architecture Master’s degree apprenticeship course. In her research work and teaching practice, Gosia explores the transdisciplinary advancement in AI and applications of machine learning in architectural theory and practice.
Notes
1 Ian Goodfellow, Yoshua Bengio, and Aaron Courville, Deep Learning (MIT Press, 2016), 421-422.
2 Luciana Parisi, Brian Massumi, and Erin Manning, Contagious Architecture: Computation, Aesthetics and Space, Illustrated edition (Cambridge, Massachusetts l London, England: MIT Press, 2013), xii.
3 Mario Carpo, The Alphabet and the Algorithm, 1st edition (Cambridge, Mass: MIT Press, 2011), 76.
4 Carpo, The Alphabet, 28.
5 Carpo, The Alphabet, 78.
6 David Eskenazi, ‘Architecture’s Digital Model Problems’, in 103rd ACSA Annual Meeting Proceedings, The Expanding Periphery and the Migrating Center, 2015,https://www.acsa-arch.org/chapter/architectures-digital-model-problems/.
7 Ian Goodfellow, Yoshua Bengio, and Aaron Courville, Deep Learning (MIT Press, 2016), 417.
8 This includes plug-ins such as LunchBoxML for Grashopper and Dynamo with a range of generalised supervised and unsupervised machine learning tools. For more information see Nate, ‘New Machine Learning Examples with LunchBoxML’, PROVING GROUND (blog), 12 March 2018,https://provingground.io/2018/03/12/new-machine-learning-examples-with-lunchboxml/.
9 ‘Christ Church: Historical Account | British History Online’, accessed 11 July 2022,https://www.british-history.ac.uk/survey-london/vol27/pp148-169.
Bibliography
Carpo, Mario. The Alphabet and the Algorithm. 1st edition. Cambridge, Mass: MIT Press, 2011.
Carpo, Mario, ‘Mario Carpo The Second Digital Turn at Talks at Google (Transcript) | PDF | Geometry | Artificial Intelligence’. Accessed 10 July 2022. shorturl.at/cgoYZ.
‘Christ Church: Historical Account | British History Online’. Accessed 11 July 2022. https://www.british-history.ac.uk/survey-london/vol27/pp148-169.
Cunningham, Pádraig, and Sarah Jane Delany. ‘Underestimation Bias and Underfitting in Machine Learning’. In Trustworthy AI - Integrating Learning, Optimization and Reasoning, edited by Fredrik Heintz, Michela Milano, and Barry O’Sullivan, 20–31. Lecture Notes in Computer Science. Cham: Springer International Publishing, 2021. https://doi.org/10.1007/978-3-030-73959-1_2.
Eskenazi, David. ‘Architecture’s Digital Model Problems’. In 103rd ACSA Annual Meeting Proceedings, The Expanding Periphery and the Migrating Center, 2015, 634-640. shorturl.at/flpX8.
Goodfellow, Ian, Yoshua Bengio, and Aaron Courville. Deep Learning. MIT Press, 2016.
Parisi, Luciana, Brian Massumi, and Erin Manning. Contagious Architecture: Computation, Aesthetics and Space. Illustrated edition. Cambridge, Massachusetts l London, England: MIT Press, 2013.
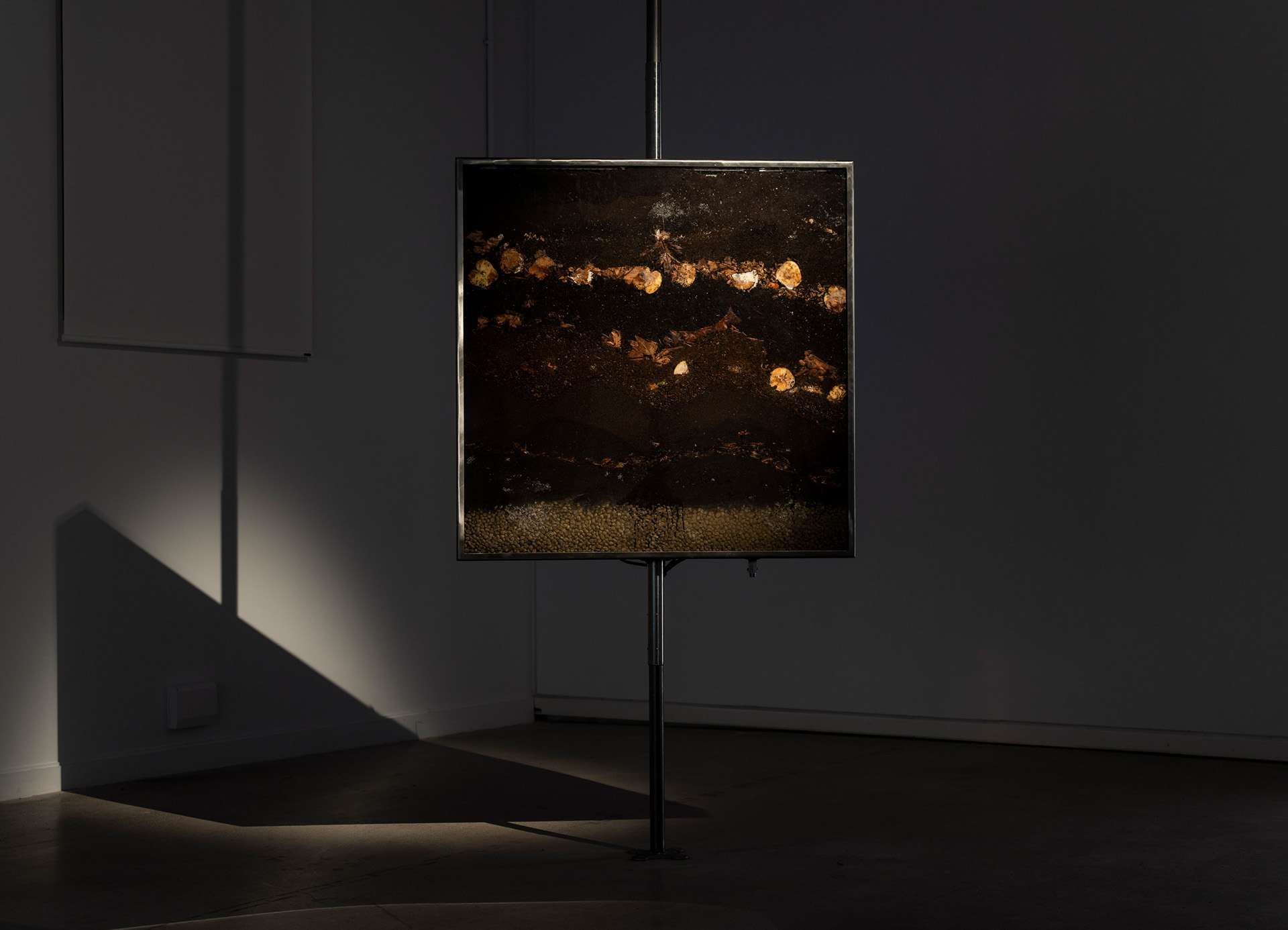